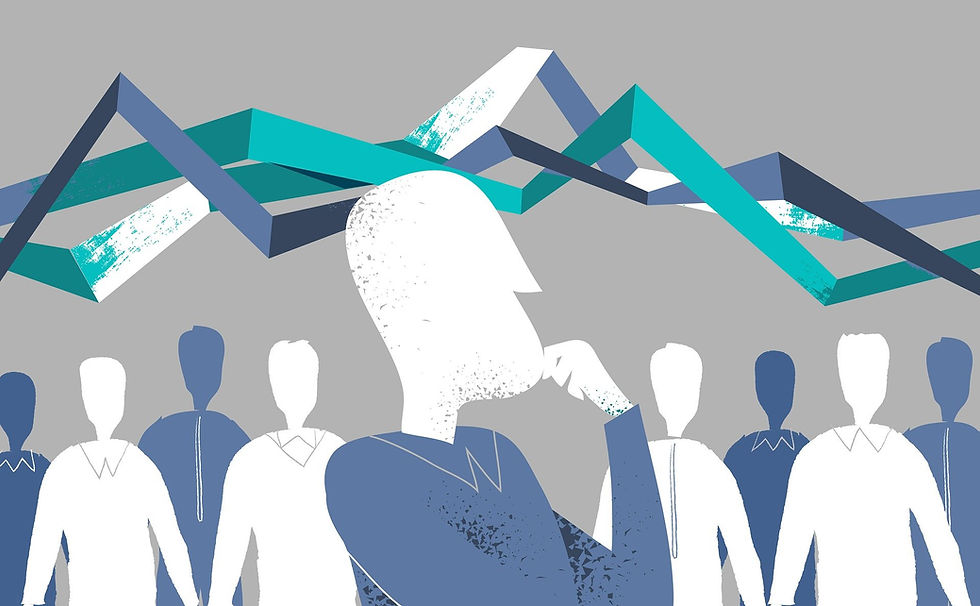
Surveys and polls are indispensable for understanding customer needs, employee sentiment, and broader market trends. However, gathering responses is only the beginning of the data journey. To transform survey data into valuable insights, organisations need to employ robust analytical techniques and tools that allow them to go beyond basic response percentages and dive into deeper, more meaningful insights.
In this post, we explore some advanced survey analytics techniques—including segmentation, cross-tabulation, and the application of machine learning (ML) and artificial intelligence (AI) for predictive insights—that can elevate your survey results from simple feedback to strategic data assets.
Segmentation: Analysing Subgroups for Nuanced Insights
Segmentation is dividing your survey respondents into distinct groups based on shared characteristics, such as demographics, purchase behaviour, or job function. This enables organisations to identify unique patterns and trends within specific segments that may not be visible in aggregate data.
For example, a customer satisfaction survey may show that satisfaction is generally high. However, by segmenting responses by age, you might discover that younger customers are less satisfied with the user experience than older customers. Armed with this information, you can implement targeted improvements aimed at increasing satisfaction among this segment.
How to Segment Effectively:
Use respondent characteristics collected during the survey, such as age, location, or department, as segmentation factors.
Use tools like Google Forms, SurveyMonkey, or more advanced platforms such as Qualtrics, which offer built-in segmentation features to create tailored reports by subgroup.
Analyse each segment independently and compare trends across groups to develop a more comprehensive understanding of your data.
Segmentation reveals deeper insights and ensures that your data-driven actions are not only impactful but also highly relevant to the groups they affect.
Cross-Tabulation: Exploring Relationships Between Variables
Cross-tabulation allows you to examine the relationship between two or more survey variables, helping you identify trends or associations that may not be apparent in the overall data. Cross-tabs are particularly useful when analysing categorical data, such as age groups or regions, in relation to specific responses.
For instance, let’s say you conducted an employee engagement survey where you asked about both job satisfaction and career development opportunities. By using cross-tabulation, you could analyse how responses to these two questions intersect. Perhaps you’ll find that employees who feel their career development needs are unmet also report lower job satisfaction levels. This insight could guide your team towards developing new career growth programmes that could positively impact overall engagement.
How to Use Cross-Tabulation:
Start with tools that offer cross-tabulation functionalities, such as Microsoft Excel or dedicated survey platforms like SurveyMonkey or Zoho Survey.
Create a matrix that compares two or more variables (e.g., satisfaction scores by department).
Look for trends, outliers, or correlations that can inform targeted strategies.
Cross-tabulation is invaluable for revealing patterns in survey data and helping organisations make connections that can drive meaningful improvements.
Machine Learning (ML) and Artificial Intelligence (AI) for Predictive Insights
In recent years, ML and AI have transformed the field of data analytics, including survey analytics. By leveraging these technologies, organisations can go beyond understanding current trends to predicting future outcomes. Predictive analytics can, for instance, forecast which employees are likely to disengage based on survey feedback patterns, or identify customer segments that may be at risk of churning.
Machine learning algorithms are particularly adept at identifying patterns in large datasets that would be difficult or impossible to spot manually. Natural Language Processing (NLP), a branch of AI, is also useful for analysing open-ended survey responses. By using NLP, organisations can classify responses into themes, gauge sentiment, and identify areas that need improvement based on feedback.
Practical Applications of ML and AI in Survey Analytics:
Sentiment Analysis: Tools like MonkeyLearn or IBM Watson use NLP to analyse the sentiment behind open-ended responses, classifying feedback as positive, neutral, or negative.
Predictive Modelling: Platforms like Google Cloud’s AutoML or DataRobot can help organisations build predictive models to anticipate future trends. For example, predicting customer satisfaction scores based on historical survey data can enable proactive improvements.
Clustering and Pattern Recognition: Clustering algorithms can group respondents with similar feedback patterns, enabling organisations to identify subgroups with specific needs or opinions.
Integrating ML and AI into survey analytics allows organisations to move from descriptive analysis to predictive and even prescriptive analytics, enabling them to make data-driven decisions that anticipate future trends.
Choosing the Right Tools for Survey Analytics
To unlock the potential of these techniques, choosing the right tools is crucial. Many survey platforms now offer advanced analytics features, making it easier than ever to apply these techniques to survey data. Below are some recommended tools and their applications:
Qualtrics: Known for its advanced analytics capabilities, including segmentation, cross-tabulation, and predictive analytics.
SurveyMonkey: Provides useful cross-tabulation features, basic sentiment analysis, and integrates well with analytics platforms like Tableau for deeper insights.
Google Forms with Google Analytics: Google Forms, combined with Google Analytics, allows for robust data collection and segmentation.
Zoho Survey and Zoho Analytics: An excellent combination for segmentation, cross-tab analysis, and more detailed reporting.
These tools vary in sophistication, so selecting the right one depends on your organisation’s specific requirements and the complexity of your survey analysis needs.
Conclusion
Survey analytics, when conducted with the right tools and techniques, enables organisations to go far beyond surface-level insights. By implementing advanced techniques such as segmentation, and cross-tabulation, and leveraging the predictive power of AI and ML, companies can extract deeper, more granular insights that drive meaningful change. These insights allow businesses to make informed, proactive decisions that can improve customer satisfaction, boost employee engagement, and foster growth.
At Synapse Junction, we specialise in helping organisations leverage survey data to its fullest potential. Whether you’re just starting with survey analytics or looking to integrate machine learning into your data processes, we can guide you through every step. Reach out to our team to explore how we can turn your survey data into strategic, actionable insights.
Comments