Future Trends in ETLT
- Synapse Junction
- Aug 1, 2024
- 4 min read
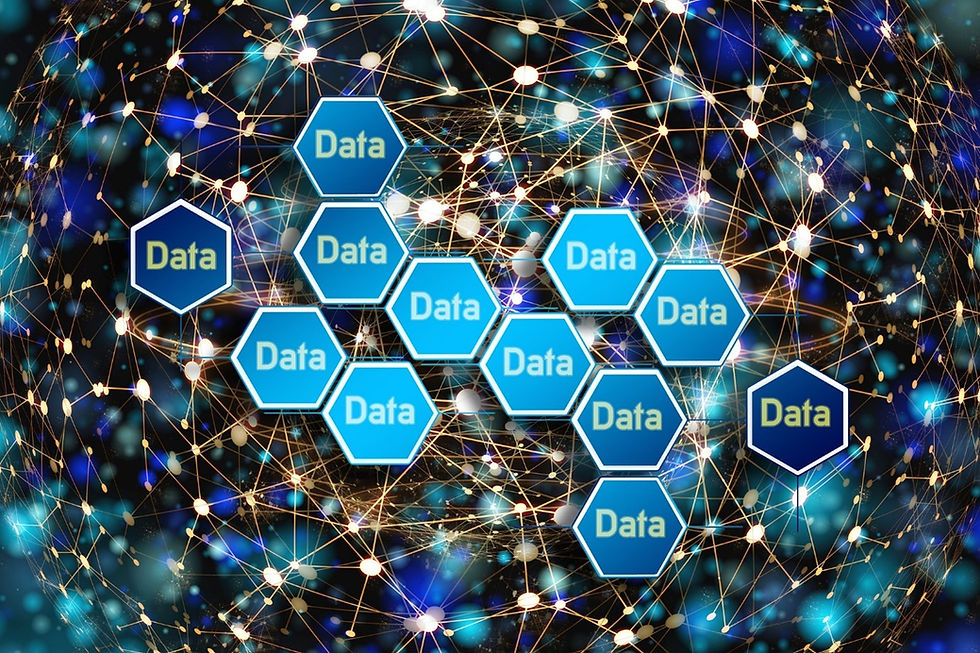
As the volume, variety, and velocity of data continue to grow, so too does the complexity of data integration and transformation processes. ETLT (Extract, Transform, Load, and Transform) has evolved as a robust approach to managing these complexities. In this blog post, we will explore future trends in ETLT, examining how emerging technologies and evolving business needs are shaping the landscape of data integration and analytics.
1. Artificial Intelligence and Machine Learning Integration
Artificial Intelligence (AI) and Machine Learning (ML) are transforming data analytics by enabling advanced predictive analytics and automated decision-making. Integrating AI and ML into ETLT processes is poised to revolutionise data transformation and analysis.
Trends
Automated Data Transformation: AI and ML can automate complex data transformations, reducing manual intervention and improving efficiency. These technologies can learn from historical data to suggest or perform transformations.
Predictive Analytics: Integrating ML models into ETLT pipelines allows for real-time predictive analytics. For example, sales data can be transformed and analysed to forecast future trends.
Anomaly Detection: AI-driven anomaly detection can identify and correct data quality issues during the transformation process, ensuring cleaner and more reliable data.
Example
Using ML models to predict customer churn rates by transforming and analysing customer interaction data in real-time.
2. Edge Computing
Edge computing involves processing data closer to its source rather than relying solely on centralised data centres. This approach is particularly relevant for IoT (Internet of Things) and real-time data analytics.
Trends
Localised Data Transformation: Performing initial data transformations at the edge reduces latency and bandwidth usage. This is crucial for applications requiring real-time insights.
Distributed ETLT Pipelines: Combining edge and cloud computing to create distributed ETLT pipelines enhances scalability and resilience. Data is pre-processed at the edge and further transformed in the cloud.
Security and Privacy: Edge computing can enhance data privacy by keeping sensitive data local and only sending aggregated or anonymised data to central servers.
Example
Smart cities using edge computing to transform and analyse traffic data in real-time, optimising traffic flow and reducing congestion.
3. Cloud-Native ETLT Solutions
Cloud-native ETLT solutions are designed to leverage the full potential of cloud computing, offering scalability, flexibility, and cost-efficiency.
Trends
Serverless Architectures: Serverless ETLT solutions, such as AWS Lambda or Azure Functions, allow for automatic scaling and pay-as-you-go pricing models. This reduces the need for managing infrastructure.
Containerisation: Using containers (e.g., Docker, Kubernetes) to deploy ETLT pipelines enhances portability and scalability across different cloud environments.
Integration with Cloud Data Warehouses: Cloud-native ETLT tools seamlessly integrate with cloud data warehouses like Amazon Redshift, Google BigQuery, and Snowflake, enabling efficient data transformation and analysis.
Example
A retail company using serverless ETLT to process and transform e-commerce data, scaling automatically during peak shopping periods.
4. DataOps and Automation
DataOps, an extension of DevOps, focuses on improving the speed and quality of data analytics through automation and collaboration. It emphasises continuous integration and delivery of data.
Trends
Pipeline Automation: Automating the entire ETLT pipeline, from data extraction to final transformation, reduces errors and accelerates data processing.
Collaborative Data Workflows: Tools that facilitate collaboration among data engineers, analysts, and business users improve the efficiency and effectiveness of ETLT processes.
Monitoring and Observability: Advanced monitoring and observability tools provide real-time insights into ETLT pipelines, helping to quickly identify and resolve issues.
Example
A financial services firm implementing DataOps practices to automate and monitor their ETLT pipelines, ensuring timely and accurate delivery of financial reports.
5. Blockchain Technology
Blockchain technology, known for its decentralised and immutable nature, is emerging as a solution for ensuring data integrity and transparency in ETLT processes.
Trends
Data Provenance and Lineage: Blockchain can provide a tamper-proof record of data transformations, ensuring transparency and traceability. This is particularly important for regulatory compliance.
Decentralised Data Processing: Using blockchain to decentralise data processing and storage enhances security and resilience against data breaches.
Smart Contracts: Automating data transformation rules and quality checks using smart contracts ensures that data integrity is maintained throughout the ETLT process.
Example
A healthcare provider using blockchain to maintain a secure and transparent record of patient data transformations, ensuring compliance with data privacy regulations.
6. Enhanced Data Governance and Privacy
As data privacy regulations become more stringent, ETLT processes must adapt to ensure compliance and protect sensitive information.
Trends
Data Anonymisation and Masking: Advanced techniques for data anonymisation and masking ensure that sensitive information is protected during and after transformation.
Compliance Automation: Automating compliance checks within ETLT pipelines ensures that data processing adheres to regulations like GDPR and CCPA.
Metadata Management: Enhanced metadata management tools track data lineage and transformations, providing transparency and accountability.
Example
A multinational corporation automating GDPR compliance checks within their ETLT pipelines to protect customer data and avoid regulatory penalties.
The future of ETLT is shaped by technological advancements and evolving business needs. Integrating AI and ML, leveraging edge computing, adopting cloud-native solutions, embracing DataOps, exploring blockchain technology, and enhancing data governance are key trends that will define the next generation of ETLT processes. By staying ahead of these trends, organisations can unlock new opportunities, improve data quality, and drive more informed decision-making.
Need guidance on how to take your ETLT process to the next level?
At Synapse Junction, we partner with you to unlock the stories hidden in your data! Contact us today to discover how to take your ETLT transformations to the next level. Unleash the power of your data with Synapse Junction, where data-driven insights become actions!
Comentários