Today's digital-first world revolves around data. Information is at our fingertips, and businesses worldwide are leveraging this data to gain valuable insights, drive decision-making, and create innovative products and services. However, the process of gathering, managing, and analysing this data is evolving. This evolution is signified by the shift from traditional data management and analytics approaches to modern practices collectively referred to as Data Operations or DataOps.
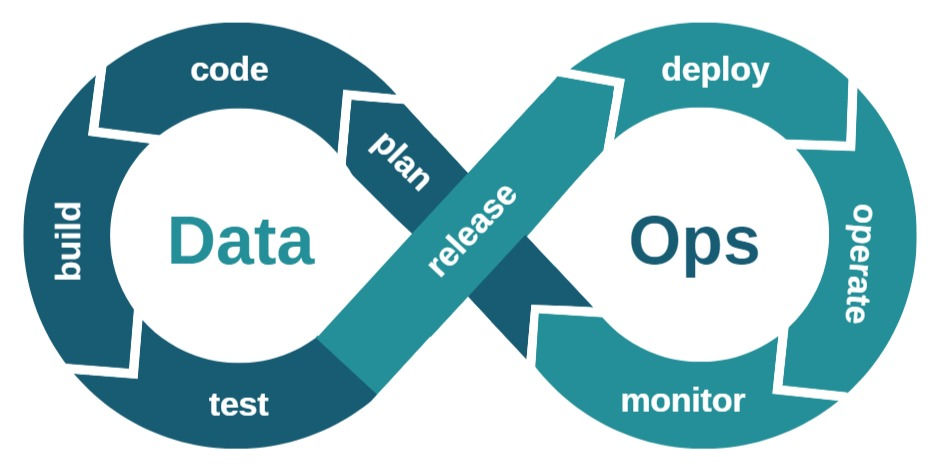
In this article, we'll delve into the world of DataOps and compare it with traditional approaches to data and analytics. We'll discuss the distinct advantages of DataOps and how it's changing the data analytics landscape for the better.
Traditional Approach to Data and Analytics: The Waterfall Method
Historically, businesses relied heavily on the traditional waterfall approach to data analytics. This approach is characterised by a linear sequence of steps, from gathering requirements and designing systems to implementation and maintenance. Each stage relies heavily on the completion of the previous one, resulting in long project cycles and delayed time-to-insight. Additionally, due to its rigid structure, the waterfall method is not adept at handling changes in requirements or unanticipated errors, leading to costly adjustments and potentially compromised data quality.
The waterfall approach also often results in siloed work, where communication gaps between teams can lead to misunderstandings and costly mistakes. The model's lack of flexibility and adaptability can stifle innovation and impede the ability to respond rapidly to changing business needs.
In today's fast-paced, data-driven world, these drawbacks are particularly problematic. Businesses require agility, speed, and collaborative environments to fully leverage their data assets, which is where DataOps comes into play.
DataOps: A Game-Changer in Data Management and Analytics
DataOps is a modern methodology that aims to improve the speed, quality, and reliability of data analytics. It brings together practices from Agile development, DevOps, and Lean Manufacturing to create an iterative, efficient, and high-quality data analytics pipeline.
Unlike the linear waterfall approach, DataOps promotes a highly collaborative, iterative, and incremental process. Agile methodologies lie at the heart of DataOps, encouraging regular reassessments of project priorities, quick adaptation to evolving requirements, and shorter development cycles, known as "sprints".
DevOps practices in DataOps automate and streamline the deployment of analytics, drastically reducing the time from development to deployment and improving overall product quality. The use of lean manufacturing principles in DataOps aids in minimising waste, improving efficiency, and maintaining a constant focus on quality, transforming the data pipeline into a "data factory".
DataOps is much more than just DevOps for data; it includes other methodologies tailored to address the unique challenges of managing enterprise-critical data operations. It targets problems such as slow analytics cycle time, lack of collaboration within data teams, rigid data architectures, and other bottlenecks.
The Promise of DataOps Automation and Observability
DataOps incorporates automation and observability, two critical aspects that supercharge its effectiveness. DataOps automation aims to manage the creation, deployment, and execution of analytics. It ensures rapid setup of workspaces, automated deployment, orchestrates the data pipeline, and fosters collaboration within teams. This streamlined workflow minimises unplanned work, reduces errors, and facilitates the swift development and deployment of analytics.
On the other hand, DataOps observability offers real-time monitoring, testing, alerting, and analysing of the data landscape. This feature provides an all-encompassing view of every data journey from source to insights. DataOps observability allows for the immediate detection, localization, and understanding of problems, enabling quick and effective resolution.
DataOps vs Traditional Approaches: A Comparative Outlook
Comparing DataOps with traditional approaches underscores the transformative shift happening in the world of data and analytics.
Agility and Speed
Unlike traditional methods that often involve long project cycles, DataOps prioritises agility and speed. With its short, iterative cycles, teams can quickly pivot as needed, adapting to new requirements and changes in real time.
Quality and Reliability
DataOps emphasises high-quality, reliable analytics. By implementing DevOps principles and rigorous testing procedures, DataOps aims to deliver error-free results, enhancing overall data quality and reliability.
Collaboration and Communication
DataOps fosters a culture of collaboration and open communication. This culture breaks down silos, promoting synergy between teams and facilitating the exchange of ideas and information.
Observability and Automation
DataOps brings in a high level of automation and observability. It provides end-to-end visibility of data flows, enabling real-time monitoring and troubleshooting. Automation eliminates manual errors and speeds up the analytics cycle time, ensuring consistent and efficient data operations.
In essence, while traditional approaches to data and analytics have their merits, the dynamic, collaborative, and automated nature of DataOps is better suited to today's fast-paced, data-driven landscape. With DataOps, businesses can leverage their data assets more effectively, achieve faster time-to-insight, and adapt swiftly to changing business needs.
The Path Forward
As we propel deeper into the digital age, where data is more than just numbers but a driver of decision-making and innovation, an agile and efficient methodology is required. That's precisely what DataOps offers.
As a business leader, understanding and implementing DataOps can lead to more informed decisions, better project management, and a more streamlined workflow. It also helps foster a culture of collaboration and continuous learning within your teams, making your organisation a dynamic powerhouse ready to tackle the challenges of the future.
For data professionals, DataOps isn't just a new framework; it's a lifeline. It ensures you stay relevant in an ever-changing landscape, updating your skill set and enhancing your career trajectory. It offers a new perspective on data management and analytics, allowing you to break free from traditional, linear methods and embrace a more iterative, responsive approach.
As data enthusiasts, incorporating DataOps principles can help make sense of the vast, complex world of data. It helps transform raw information into meaningful insights faster and more efficiently, making your journey with data a more fruitful and satisfying one.
But DataOps isn't just about immediate benefits. Its adoption signifies a strategic, forward-thinking approach to managing data. As the volume and complexity of data increase exponentially, businesses that adopt DataOps today will be better equipped to navigate the future. They will be ready to leverage the opportunities that advanced technologies, such as machine learning and artificial intelligence, bring to the table.
In essence, understanding and embracing DataOps is a crucial step towards a more agile, efficient, and high-quality data future. It represents a commitment to continuous improvement, innovation, and a relentless pursuit of excellence. By adopting DataOps, we are not just reacting to the changing landscape of data management and analytics; we're proactively shaping it. The future of data is here, and it's time we embraced it wholeheartedly. As we embark on this journey, let's remember that it's not just about handling data better; it's about building a resilient, future-ready organisation that thrives on change, cherishes innovation, and values collaboration.
Comments