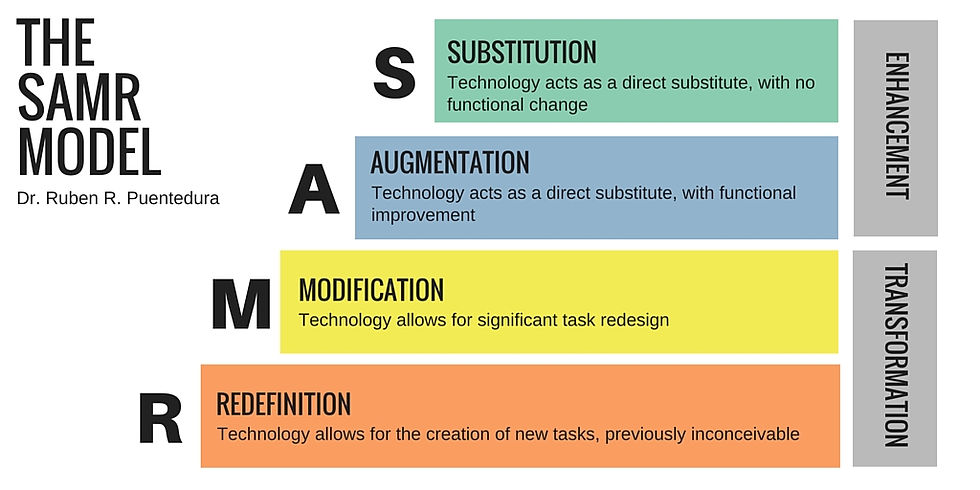
Dr Ruben Puentedura’s SAMR model—Substitution, Augmentation, Modification, and Redefinition—was originally developed to assess the integration of technology in education. However, its structured approach can be effectively applied to categorise the adoption and evolution of technology in data analytics. By leveraging this model, organisations can evaluate how AI, Machine Learning (ML), and Large Language Models (LLMs) transform data analytics processes, driving efficiency, innovation, and strategic decision-making.
The SAMR model offers four levels of technological integration:
Substitution – Technology acts as a direct tool substitute with no functional change.
Augmentation – Technology enhances a process while maintaining its core structure.
Modification – Technology significantly redesigns a process, improving its capabilities.
Redefinition – Technology enables entirely new, previously inconceivable tasks.
Applying the SAMR Model to AI, ML, and LLMs in Data Analytics
Substitution: Automating Traditional Analytical Tasks
At the substitution level, AI and ML replace manual analytical tasks without fundamentally altering workflows. Examples include:
Automating spreadsheet-based data cleaning using AI-powered macros in Microsoft Excel or Unstructured Data Warehouses.
Using ML models for simple predictive analytics in Power BI that were previously performed using traditional statistical methods.
Deploying LLMs to generate basic reports and summaries in Azure Synapse Analytics, reducing the need for manual report writing.
While these applications improve efficiency, they do not radically transform the analytical approach.
Augmentation: Enhancing Efficiency and Accuracy
At this stage, AI, ML, and LLMs enhance existing data analytics processes, providing additional functionality while preserving the original workflow structure. Examples include:
AI-assisted Data Preparation: Power Query in Excel and Power BI uses AI to suggest data transformations and cleaning steps automatically.
Natural Language Processing (NLP) for Queries: The Power BI Q&A feature enables analysts to interact with datasets using natural language, improving accessibility.
Enhanced Visualisation: AI-driven Power BI Insights dynamically adapt to user queries, offering deeper insights than static reports.
Here, technology improves productivity, accuracy, and accessibility while keeping traditional processes intact.
Modification: Transforming Data Analytics Workflows
Modification represents a significant shift in how data analytics is conducted, leveraging AI and ML to reshape workflows. This level includes:
Real-time Anomaly Detection: Azure Synapse Analytics leverages ML models to continuously monitor data streams, identifying outliers far more effectively than rule-based systems.
Automated Data Storytelling: Power BI's Smart Narrative feature generates complex narratives that provide context and insights beyond static reports.
Adaptive Decision Support Systems: AI-powered Power Automate workflows guide business strategies based on evolving data patterns by integrating real-time insights.
At this stage, AI and ML are not merely enhancing traditional processes; they are reconfiguring how insights are derived and acted upon.
Redefinition: Pioneering New Analytical Capabilities
Redefinition represents the pinnacle of AI and ML integration, enabling tasks that were previously impossible. This level includes:
AI-driven Autonomous Analytics: Azure Machine Learning enables automated model training and deployment, allowing businesses to generate insights without manual intervention.
Conversational Analytics with LLMs: Copilot in Microsoft 365 and Azure OpenAI Service facilitate natural conversations with complex datasets, empowering non-technical users to extract deep insights.
Synthetic Data Generation: AI models in Azure AI create high-fidelity synthetic data for training, testing, and privacy-preserving analytics, unlocking new possibilities for data-driven innovation.
Strategic Implications for Data Leaders
For organisations at the forefront of data and analytics, the SAMR model provides a structured lens to evaluate AI adoption and maturity. While substitution and augmentation offer efficiency gains, long-term competitive advantage lies in modification and redefinition. Data leaders should:
Assess Current AI Maturity: Identify where existing AI and ML initiatives fit within the SAMR framework.
Develop a Strategic Roadmap: Plan a transition from lower levels to modification and redefinition.
Invest in Talent and Infrastructure: Ensure teams possess the skills and resources to leverage advanced AI capabilities.
Conclusion
By applying the SAMR model to AI, ML, and LLMs in data analytics, organisations can systematically assess their technological evolution. While initial AI adoption may begin with basic automation, true value emerges when AI redefines analytical capabilities, enabling new ways to extract, interpret, and act upon data. As the field advances, data leaders must not only embrace these innovations but also strategically harness them to drive meaningful transformation.
At Synapse Junction, we specialise in guiding organisations through their AI, ML, and LLM journeys, ensuring they harness these technologies effectively to unlock the full potential of their data. Whether you're looking to enhance efficiency, transform workflows, or redefine analytics capabilities, our experts are here to help. Partner with us to take your data analytics to the next level.
Comments